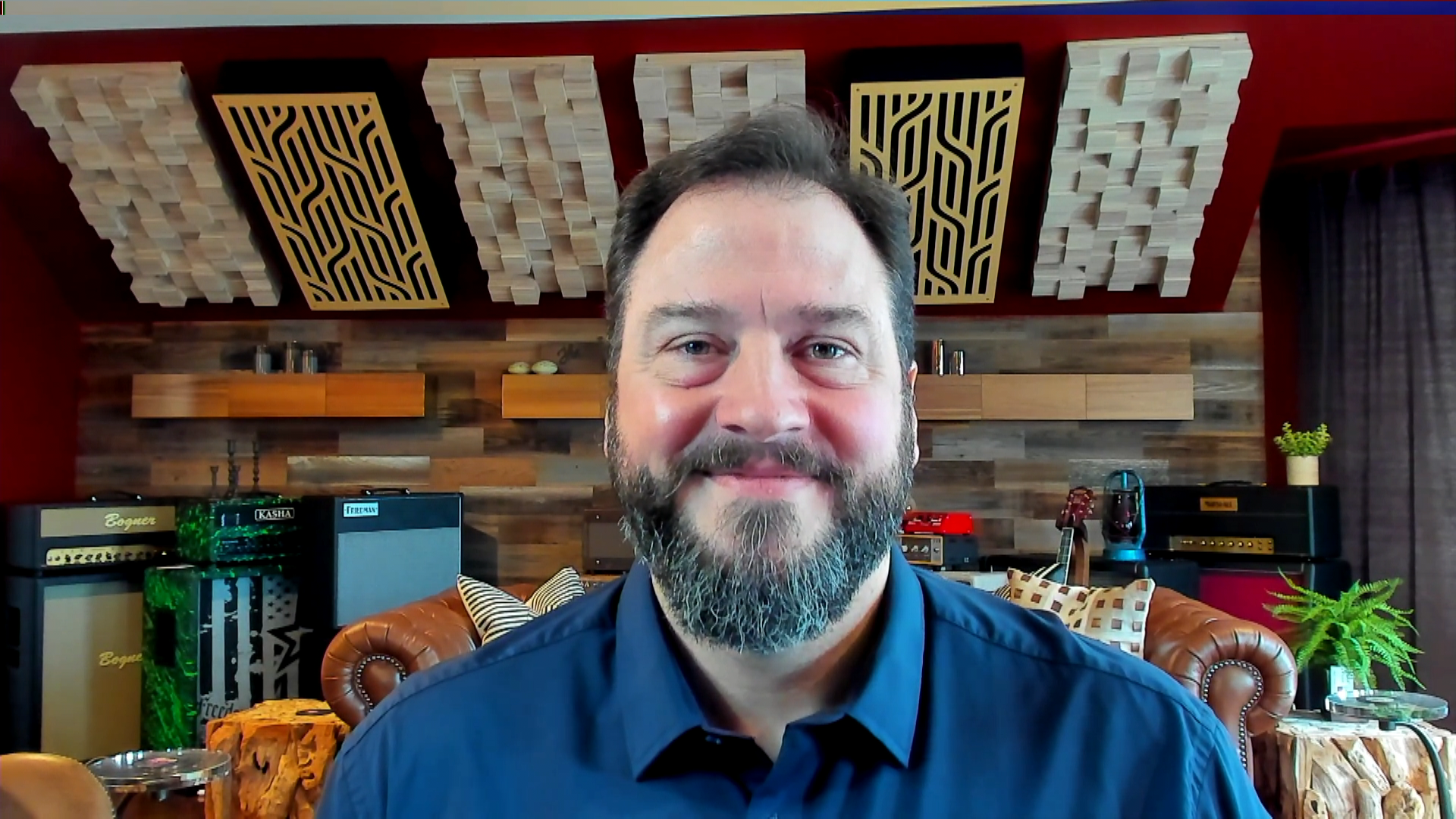
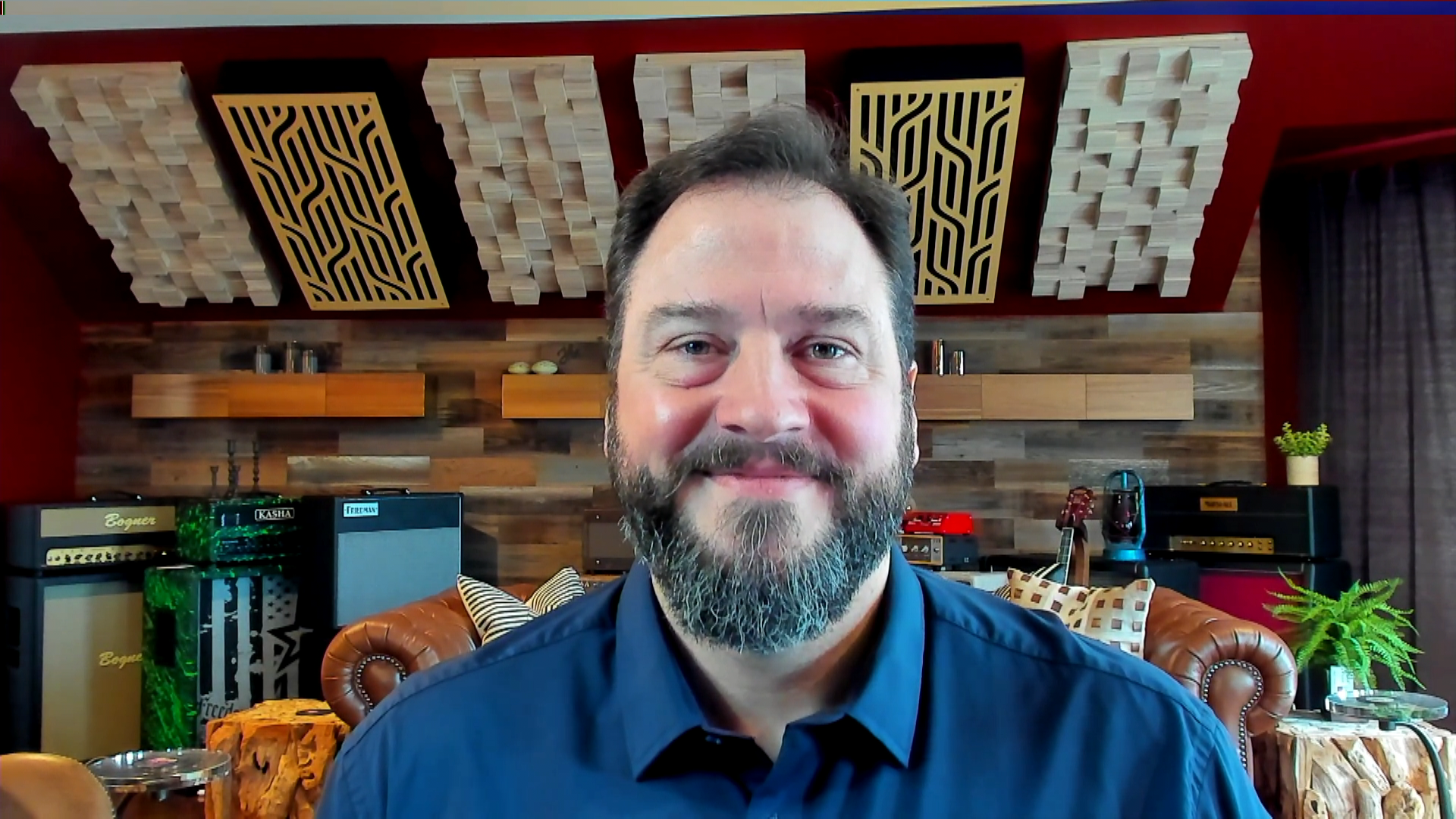
With the advancement of cloud and artificial intelligence technologies, organizations are asking more of their data than ever.
With use cases such as computer vision and natural language processing heavily relying on foundational AI, Neuralmagic Inc. has inserted itself into the value area of optimizing and scaling machine learning models.
“These big, generational models or foundational models, as we’re calling them, are great,” said Jay Marshall (pictured), head of global business development at Neuralmagic. “But enterprises want to do that with their data, on their infrastructure, at scale and at the edge. We’re helping enterprises accelerate that through optimizing models and then delivering them at scale in a more cost-effective fashion.”
Marshall spoke with theCUBE industry analyst John Furrier at the AWS Startup Showcase: “Top Startups Building Generative AI on AWS” event, during an exclusive broadcast on theCUBE, SiliconANGLE Media’s livestreaming studio. They discussed how companies are leveraging AI/ML to deploy applications at scale and how the company provides aid in that regard. (* Disclosure below.)
Companies that operate using machine learning technologies have to carry out three requisite processes: the building, training and deployment of models. As these processes advance in intricacy and scale, the cost requirements continue to mount. Thus, Neuralmagic is focusing on delivering operational efficiency with a potent mix of in-house and open-source technologies.
“They want to do it, but they don’t really know where to start,” Marshall explained. “So for that situation, we actually have an open-source toolkit that can help you get into this optimization. And that runtime, that inferencing runtime, which is purpose-built for CPUs, allows you to not have to worry about things like available hardware accelerators and integrating into the application stack.”
One major topic in current enterprise AI is the argument on a “lift and shift” adaptation of existing resources versus building everything up from scratch, which is often called “AI-native.” The major differentiator between both of these approaches is the ongoing innovations in deep learning and neural networks, according to Marshall.
“I think it’s more the folks that came up in that neural network world, so it’s a little bit more second nature,” he stated. “Whereas for some traditional data scientists starting to get into neural networks, you have the complexity there and the training overhead and a lot of the aspects of getting a model finely tuned, such as hyper parameterization, among other things.”
With either approach, the company’s goal is to abstract away that complexity in deployment so that enterprises can manage their workloads in a versatile variety of scenarios with standard infrastructure requirements.
As the lifeblood of ML models, data must be collected and consolidated into databases which, themselves, must be harnessed properly for optimal results. Purpose-built databases, such as from Amazon Web Services Inc., have emerged as the way to go for enterprise use cases, according to Marshall.
“I know that with AWS, they always talk about purpose-built databases. And I always liked that because you don’t have one database that can do everything,” he said. “Even with the ones that say they can, you still have to account for implementation detail differences.”
In terms of presenting itself to end users, Neuralmagic’s primary strength is on the training side of things. And the company’s strategic set of integrations with other partner tools extend further across the MLOps pipeline.
“I think where we hook customers in lies specifically in the training side,” he said. “For those that want to really optimize a model and handle deployment, we run that optimized model. That’s where we’re able to provide. We even have a “Labs” offering in terms of being able to pair up our engineering teams with a customer’s engineering teams, and we can actually help with most of that pipeline.”
Another area of expertise that sets the company apart is sparsification, an optimization approach for ML models, Marshall added. Within a model, it removes redundant components and information from an over-parameterized network.
“I think, in general, any neural network can be sparsified,” he said. “It’s an ML optimization process that we specialize in for situations where you’re trying get AI into production, you have cost or performance-related concerns.”
Here’s the complete video interview, part of SiliconANGLE’s and theCUBE’s coverage of the AWS Startup Showcase: “Top Startups Building Generative AI on AWS” event:
(* Disclosure: Neuralmagic Inc. sponsored this segment of theCUBE. Neither Neuralmagic nor other sponsors have editorial control over content on theCUBE or SiliconANGLE.)
THANK YOU