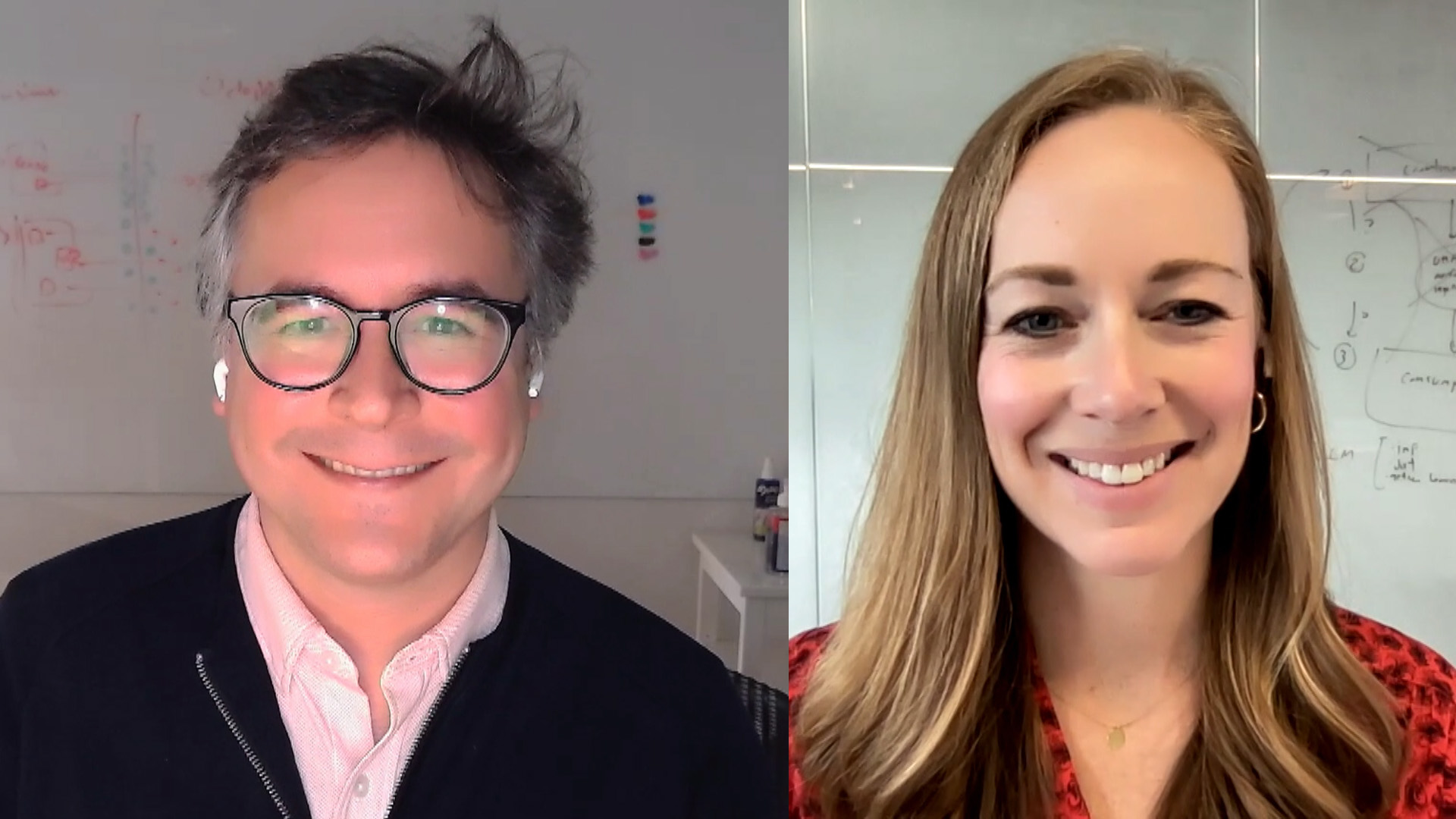
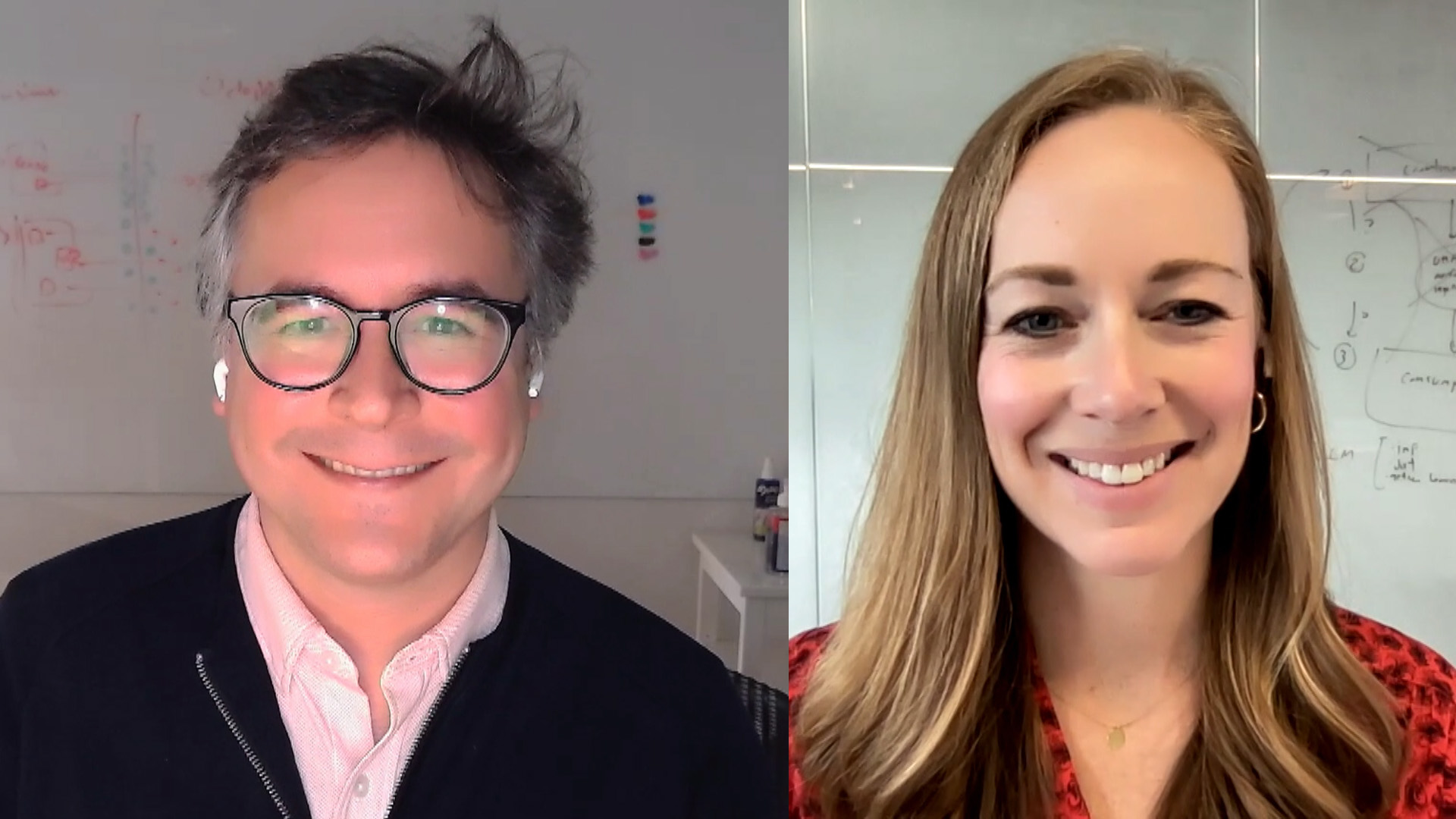
With big disruptors like ChatGPT and Bing giving the world a glimpse of what’s possible with artificial intelligence at full strength, companies globally are joining the trend of becoming artificial intelligence-native and operationalizing machine learning models.
But, like the cloud’s early days, several costs associated with training and deploying ML models aren’t immediately obvious. So, companies such as OctoML Inc. are digging beyond the surface to help users deploy the most performant but cost-effective model possible in production, according to Luis Ceze (pictured, left), co-founder and chief executive officer of OctoML.
“Given that model cost grows directly with model usage, what you want to do is make sure that once you put a model into production, you have the best cost structure possible so that you’re not surprised when it gets popular,” Ceze said. “It’s important for us to keep in mind that generative AI models like ChatGPT are huge, expensive energy hogs and cost a lot to run.”
Ceze and Anna Connolly (right), vice president of customer success and experience at OctoML, spoke with theCUBE industry analyst John Furrier at the AWS Startup Showcase: “Top Startups Building Generative AI on AWS” event, during an exclusive broadcast on theCUBE, SiliconANGLE Media’s livestreaming studio. They discussed the need to address the cost aspect in the AI equation. (* Disclosure below.)
The two major expenditure areas with machine learning can be split broadly into production and training. And while training costs are large, up-front outlays, production costs are fractional but build up over time and with increased usage.
“I think we are increasingly going to see the cost of production outpacing the cost of training by a lot,” Connolly explained. “People talk about training costs now because that’s what they’re confronting now, because they’ve been so focused on getting models performant enough to even use in an application. And now that we have them and they’re that capable, we’re really going to start to see production costs go up a lot.”
In essence, as these generative ML models become increasingly intricate, the requirements to keep them running at scale will almost certainly surpass that of training, Ceze believes.
“Let me give you an example,” he explained. “If you have a model that costs, say, $1 to $2 million to train, but then it costs about one or two cents per session to use it … if you have a million active users, even if they use it just once a day, it’s 10 to $20,000 a day to operate that model in production.”
Latency is an area where companies can shave off considerable costs. This is especially true at the higher levels, where paying for reduced latency begins to yield diminishing marginal returns on spending, according to Ceze.
“Making it faster won’t make a measurable difference in experience, but it’s gonna cost a lot more,” he stated. “What we should think about is throughput per dollar and to understand that what you want here is the highest throughput per dollar, which may come at the cost of higher latency.”
While there’ll always be costs associated with running an ML model at scale, enterprises can take several measures to shave a few percentages and maintain a tight throughput-per-dollar ratio, according to Connolly. The first of them is streamlining the deployment process so that minimal engineering is required to get the application running in the first place. The second involves extracting more value out of already-owned compute resources.
“In making deployment easier overall, there’s a lot of manual work that goes into benchmarking, optimizing and packaging models for deployment,” Connolly said. “Because the performance of machine learning models can be really hardware dependent, you have to go through this process for each target you want to consider running your model on.”
Optimizing large ML models at scale often implies a long, laborious process of efficiently matching data and software to existing hardware based on scale and performance needs. Given its experience, OctoML helps enterprises figure out that sweet spot.
“We do see customers who leave money on the table by running models that haven’t been optimized specifically for the hardware target they’re using,” Ceze said. “For some teams, they just don’t have the time to go through an optimization process, whereas others might lack kind of specialized expertise. And this is something we can bring.”
Here’s the complete video interview, part of SiliconANGLE’s and theCUBE’s coverage of the AWS Startup Showcase: “Top Startups Building Generative AI on AWS” event:
(* Disclosure: OctoML Inc. sponsored this segment of theCUBE. Neither OctoML nor other sponsors have editorial control over content on theCUBE or SiliconANGLE.)
THANK YOU